Mastering Image Annotation for Machine Learning
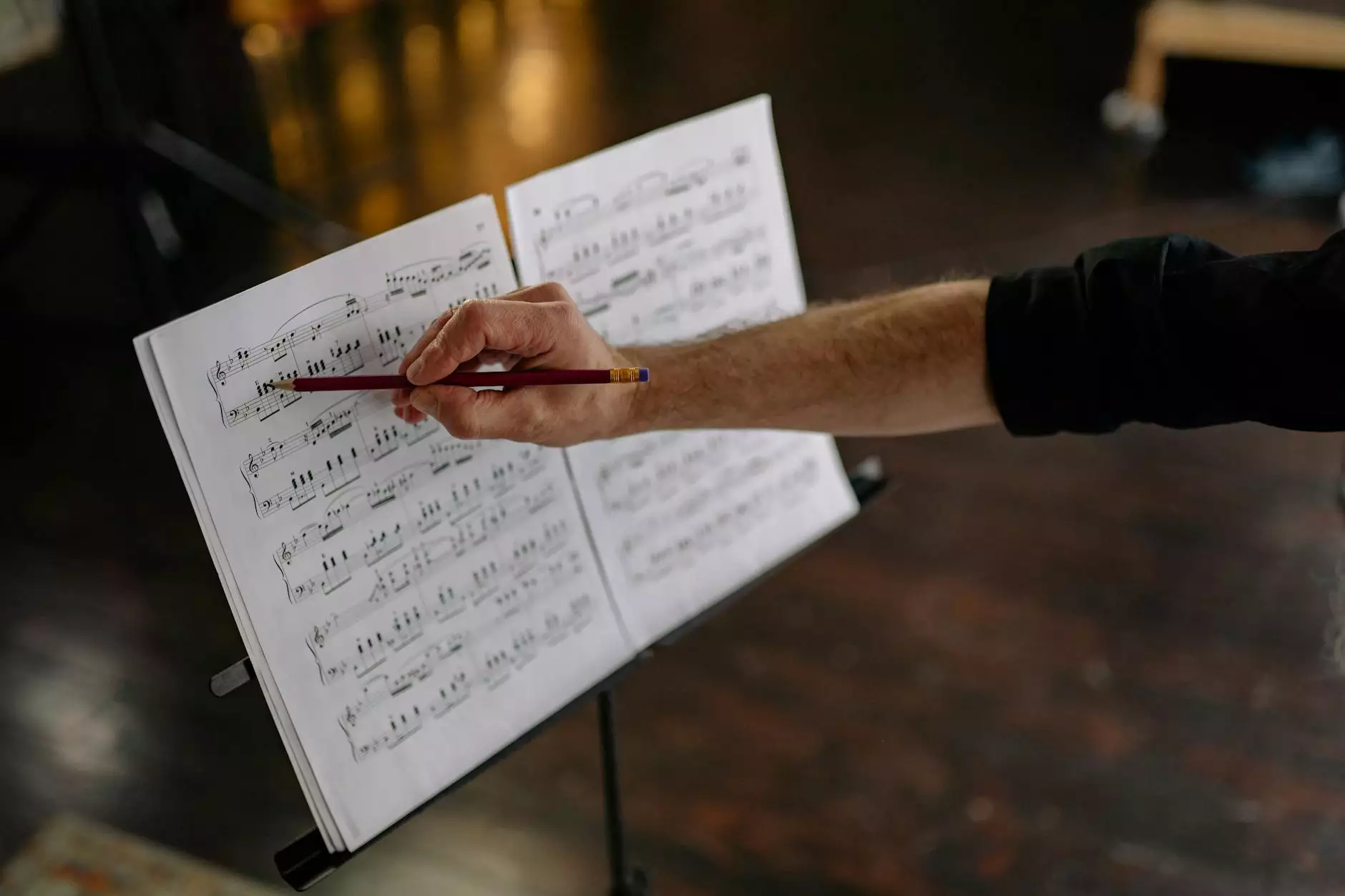
Image annotation for machine learning is a crucial process that significantly impacts the performance of artificial intelligence models. As the demand for AI solutions continues to grow across various industries, understanding the intricacies of image annotation becomes paramount. In this comprehensive guide, we will delve into the depths of image annotation, discussing its importance, types, tools, and how businesses like Keylabs.ai can leverage data annotation effectively.
Understanding Image Annotation
Image annotation involves labeling or tagging images with metadata. This process is essential for teaching machines to understand visual data. By applying image annotation for machine learning, AI models can recognize, classify, and interpret images accurately. Without proper annotation, the data fed into the models could lead to erroneous conclusions, undermining the AI's utility.
The Importance of Image Annotation in Machine Learning
The importance of image annotation cannot be overstated. Here are some key reasons why it’s critical:
- Improved Accuracy: Accurate annotations lead to more reliable AI outputs.
- Training Data Quality: The quality of training data directly impacts model performance.
- Automation and Scalability: Properly annotated images enable scalable AI solutions that can automate complex processes.
- Enhanced User Experience: Applications powered by well-trained AI provide a better user experience.
Types of Image Annotation Techniques
When it comes to image annotation for machine learning, several techniques are employed. Each technique serves a unique purpose depending on the intended AI application. Here are some of the most common types of image annotation:
1. Bounding Box Annotation
This technique entails drawing a rectangular box around an object of interest within an image. It's commonly used in object detection tasks. For instance, when training models to identify cars in traffic images, bounding boxes can precisely outline each car.
2. Polygon Annotation
Polygon annotation involves creating irregular shapes to outline objects more precisely than bounding boxes. This method is particularly useful for segmenting images where the objects have complex shapes, such as outlined streets in satellite images.
3. Semantic Segmentation
In semantic segmentation, each pixel is classified into a category, allowing for detailed analysis. This technique is vital in applications like medical imaging, where differentiating between various tissues can be critical.
4. Landmark Annotation
This technique places specific points on key features within an image, which is particularly useful in facial recognition technologies. Landmarks can represent the corners of eyes, nose, and mouth in facial datasets.
5. Image Classification Annotation
Here, images are categorized into predefined classes. For example, images can be labeled as "cat," "dog," or "bird," allowing AI models to understand and classify new images correctly.
The Role of Data Annotation Tools and Platforms
Choosing the right data annotation tool or platform is essential for efficiently executing image annotation for machine learning. The quality and speed of annotations can make a significant difference in the overall performance of machine learning models. Keylabs.ai offers robust solutions in the realm of data annotation, facilitating an efficient workflow. Here’s what makes a good data annotation tool:
1. User-Friendly Interface
A good annotation tool should have an intuitive user interface, allowing annotators to work efficiently. A user-friendly environment helps minimize the learning curve for new users.
2. Collaboration Features
Collaboration tools enable teams to work on annotations simultaneously, streamlining the process and enhancing productivity. Look for platforms that offer real-time collaboration capabilities.
3. Quality Control Mechanisms
Incorporating quality assurance checks, such as consensus reviews, helps maintain high-quality annotations. This ensures that the data used for training is as accurate and reliable as possible.
4. Scalability
As your data needs grow, your annotation tool should be able to scale with it. A robust platform can handle large volumes of data without compromising performance or quality.
5. Integration Capabilities
The ability to integrate with other tools and systems is crucial. Integration allows for seamless workflows, where annotated data can be moved to machine learning frameworks effortlessly.
Best Practices in Image Annotation
To maximize the efficiency of image annotation for machine learning, follow these best practices:
1. Define Clear Guidelines
Establishing clear annotation guidelines is vital for consistency. Annotators should understand the objectives and standards to ensure that everyone is on the same page.
2. Continuous Training for Annotators
Investing in training programs for annotators can enhance their skills and knowledge. Informed annotators produce higher-quality results, reducing the need for extensive revisions.
3. Implement a Feedback Loop
Encouraging a feedback loop can help identify common issues and improve the annotation process. Incorporate feedback from both annotators and data scientists to enhance quality.
4. Leverage Automation
Utilize semi-automated annotation tools that use AI assistance to speed up the process while maintaining accuracy. Automation can help manage large datasets more efficiently.
Conclusion: The Future of Image Annotation
The landscape of image annotation for machine learning is continually evolving. With advancements in AI technologies, the demand for high-quality annotated datasets will only increase. Businesses such as Keylabs.ai are at the forefront of this evolution, providing innovative solutions to meet these needs.
As AI technologies advance, the methods and practices surrounding image annotation will adapt accordingly. Companies embarking on their AI journey must pay close attention to the quality of their data annotation processes. Doing so will ensure that their AI models achieve optimum performance, leading to better decision-making and more successful outcomes.
Incorporating an effective image annotation strategy not only enhances the functionality of machine learning models but also positions businesses for success in an increasingly competitive landscape. As the future unfolds, embracing the art and science of image annotation will undoubtedly become a cornerstone of AI innovation.
For more information and cutting-edge solutions, visit Keylabs.ai. Discover how our innovative Data Annotation Tools and Data Annotation Platforms can accelerate your AI initiatives today!